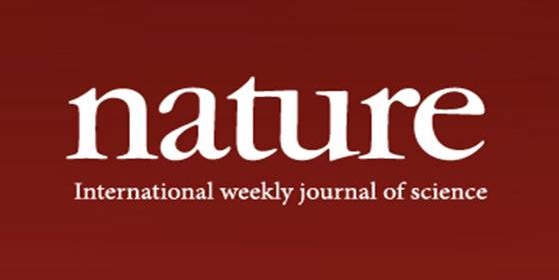
Read original story at Nature.com
Nearly 20 years ago, I was fortunate enough to play friendly blitz chess against former world champion Garry Kasparov. It was quite an experience; his competitive spirit and creative genius were palpable. I had recently founded Elixir Studios, which specialized in artificial intelligence (AI) games, and my ambition was to conduct cutting-edge research in the field. AI was on my mind that day: Kasparov had played chess against IBM’s supercomputer Deep Blue just a few years before. Now, he sets out the details of that titanic event in his memoir Deep Thinking.
The 1997 match was a watershed for AI and an extraordinary technical feat. Strangely, although Kasparov lost, it left me more in awe of the incredible capabilities of the human brain than of the machine. Kasparov was able to compete against a computational leviathan and to complete myriad other tasks that make us all distinctly human. By contrast, Deep Blue was hard-coded with a set of specialized rules distilled from chess grandmasters, and empowered with a brute-force search algorithm. It was programmed to do one thing only; it could not have played even a simpler game such as noughts and crosses without being completely reprogrammed. I felt that this brand of ‘intelligence’ was missing crucial traits such as generality, adaptability and learning.
As he details in Deep Thinking, Kasparov reached a similar conclusion. The book is his first thorough account of the match, and it offers thoughtful meditations on technology. The title references what he believes chess engines cannot do: they can calculate, but not innovate or create. They cannot think in the deepest sense. In drawing out these distinctions, Kasparov provides an impressively researched history of AI and the field’s ongoing obsession with chess.
For decades, leading computer scientists believed that, given the traditional status of chess as an exemplary demonstration of human intellect, a competent computer chess player would soon also surpass all other human abilities. That proved not to be the case. This has to do partly with differences between human and machine cognition: computers can easily perform calculation tasks that people consider incredibly difficult, but totally fail at commonsense tasks we find intuitive (a phenomenon called Moravec’s paradox). It was also due to industry and research dynamics in the 1980s and 1990s: in pursuit of quick results, labs ditched generalizable, learning-based approaches in favour of narrow, hand-coded solutions that exploited machines’ computational speed.
The focus on brute-force approaches had upsides, Kasparov explains. It may not have delivered on the promise of general-purpose AI, but it did result in very powerful chess engines that soon became popularly available. Today, anyone can practise for free against software stronger than the greatest human chess masters, enabling enthusiasts worldwide to train at top levels. Before Deep Blue, pessimists predicted that the defeat of a world chess champion by a machine would lead to the game’s death. In fact, more people play now than ever before, according to World Chess Federation figures.
Chess engines have also given rise to exciting variants of play. In 1998, Kasparov introduced ‘Advanced Chess’, in which human–computer teams merge the calculation abilities of machines with a person’s pattern-matching insights. Kasparov’s embrace of the technology that defeated him shows how computers can inspire, rather than obviate, human creativity.
In Deep Thinking, Kasparov also delves into the renaissance of machine learning, an AI subdomain focusing on general-purpose algorithms that learn from data. He highlights the radical differences between Deep Blue and AlphaGo, a learning algorithm created by my company DeepMind to play the massively complex game of Go. Last year, AlphaGo defeated Lee Sedol, widely hailed as the greatest player of the past decade. Whereas Deep Blue followed instructions carefully honed by a crack team of engineers and chess professionals, AlphaGo played against itself repeatedly, learning from its mistakes and developing novel strategies. Several of its moves against Lee had never been seen in human games — most notably move 37 in game 2, which upended centuries of traditional Go wisdom by playing on the fifth line early in the game.
Most excitingly, because its learning algorithms can be generalized, AlphaGo holds promise far beyond the game for which it was created. Kasparov relishes this potential, discussing applications from machine translation to automated medical diagnoses. AI will not replace humans, he argues, but will enlighten and enrich us, much as chess engines did 20 years ago. His position is especially notable coming from someone who would have every reason to be bitter about AI’s advances.
His account of the Deep Blue match itself is fascinating. Famously, Kasparov stormed out of one game and gave antagonistic press conferences in which he protested against IBM’s secrecy around the Deep Blue team and its methods, and insinuated that the company might have cheated. In Deep Thinking, Kasparov offers an engaging insight into his psychological state during the match. To a degree, he walks back on his earlier claims, concluding that although IBM probably did not cheat, it violated the spirit of fair competition by obscuring useful information. He also provides a detailed commentary on several crucial moments; for instance, he dispels the myth that Deep Blue’s bizarre move 44 in the first game of the match left him unrecoverably flummoxed.
Kasparov includes enough detail to satisfy chess enthusiasts, while providing a thrilling narrative for the casual reader. Deep Thinking delivers a rare balance of analysis and narrative, weaving commentary about technological progress with an inside look at one of the most important chess matches ever played.