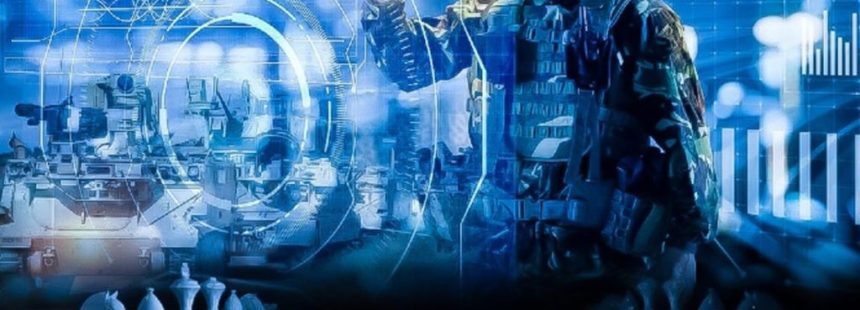
Bingo. “Future conflicts will not be won by the force with the highest computing power, most advanced chip design, or best tactical training, but by the force that most successfully employs novel algorithms to augment human decision-making.” https://t.co/Ia5QntpmLc
— Garry Kasparov (@Kasparov63) July 8, 2022
By TREVOR PHILLIPS-LEVINE, MICHAEL KANAAN, DYLAN “JOOSE” PHILLIPS-LEVINE, WALKER D. MILLS, AND NOAH SPATARO
This article is a reprint. You can read the original at War on the Rocks
“Gary Kasparov, one of the greatest chess players of all time, developed advanced chess after losing his 1997 match to IBM’s Deep Blue supercomputer. Advanced chess marries the computational precision of machine algorithms with the intuition of human beings. Similar in concept to manned-unmanned teaming or the “centaur model,” Kasparov’s experimentation has important implications for the military’s use of AI.
In 2005, a chess website hosted an advanced chess tournament open to any player. Extraordinarily, the winners of the tournament were not grandmasters and their machines, but two chess amateurs utilizing three different computers. Kasparov observed, “their skill at manipulating and ‘coaching’ their computers to look very deeply into the positions effectively counteracted the superior chess understanding of their Grandmaster opponents and the greater computational power of other participants.” Kasparov concluded that a “weak human + machine + better process was superior to a strong computer alone and … superior to a strong human + machine + inferior process.” This conclusion became known as Kasparov’s Law.
As the Department of Defense seeks to better use artificial intelligence, Kasparov’s Law can help design command-and-control architecture and improve the training of the service members who will use it. Kasparov’s Law suggests that for human-machine collaboration to be effective, operators must be familiar with their machines and know how to best employ them. Future conflicts will not be won by the force with the highest computing power, most advanced chip design, or best tactical training, but by the force that most successfully employs novel algorithms to augment human decision-making. To achieve this, the U.S. military needs to identify, recruit, and retain people who not only understand data and computer logic, but who can also make full use of them. Military entrance exams, general military training, and professional military education should all be refined with this in mind.
Building a Better Process
Kasparov’s key insight was that building a “better process” requires an informed human at the human-machine interface. If operators do not understand the rules and the limitations of their AI partners, they will ask the wrong questions or command the wrong actions.
Kasparov’s “weak human” does not mean an inept or untrained one. The “weak human” understands the computer’s rules. The two amateurs that won the 2005 chess match used their knowledge of the rules to ask the right questions in the right way. The amateurs were not Grandmasters or experts with advanced strategies. But they were able to decipher the data their computers provided to unmask the agendas of their opponents and calculate the right moves. In other words, they used a computer to fill the role of a specialist or expert, and to inform their decision-making process.
The number and type of sensors that feed into global networks is growing rapidly. As in chess, algorithms can sift, sort, and organize intelligence data in order to make it easier for humans to interpret. AI algorithms can find patterns and probabilities while humans determine the contextual meaning to inform strategy. The critical question is how humans can best be positioned and trained to do this most effectively.
Familiarity and Trust
When human operators lack familiarity with AI-enhanced systems, they often suffer from either too little or too much confidence in them. Teaching military operators how to use AI properly requires teaching them a system’s limits and inculcating just the right level of trust. This is particularly crucial in life or death situations where human operators must decide when to turn off or override AI. The level of trust given to an AI is dependent on the maturity and proven performance of a system. When AI systems are in the design or testing phases, human operators should be particularly well-versed in their machine’s limitations and behavior so they can override it when needed. But this changes as the AI becomes more reliable.
Consider the introduction of the automatic ground collision avoidance system (auto-GCAS) into F-16 fighter jets. Adoption was stinted by nuisance “pull-ups,” when the AI unnecessarily took over the flight control system during early flight testing and fielding. The distrust this initially created among pilots was entirely understandable. As word spread throughout the F-16 community, many pilots began disabling the system altogether. But as the technology became more reliable, this distrust itself became a problem, preventing pilots from taking advantage of a proven life-saving algorithm. Now, newer pilots are far more trusting. Lieutenant David Alman, an Air National Guard pilot currently in flight training for the F-16, told the authors that “I think the average B-course student hugely prefers it [auto-GCAS].” In other words, once the system is proven, there is less need to train future aircrews as thoroughly in their machine’s behavior and teach them to trust it.
It took a number of policy mandates and personnel turnovers before F-16 pilots began to fly with auto-GCAS enabled during most missions. Today, the Defense Advanced Projects Agency and the U.S. Air Force are attempting to automate parts of aerial combat in their Air Combat Evolution program. In the program, trained pilots’ trust is evaluated when teamed with AI agents. One pilot was found to be disabling the AI agent before it had a chance to perform due to their preconceived distrust of the system. Such overriding behaviors negate the benefits that AI algorithms are designed to deliver. Retraining programs may help, but if a human operator continues to override their AI agents without cause, the military should be prepared to remove them from processes that contain AI interaction.
At the same time, overconfidence in AI can also be a problem. “Automation bias” or the over-reliance on automation occurs when users are unaware of the limits of their AI. In the crash of Air France 447, for example, pilots suffered from cognitive dissonance after the autopilot disengaged in a thunderstorm. They failed to recognize that the engine throttles, whose physical positions do not matter when autopilot is on, were set near idle power. As the pilots pulled back on the control stick, they expected the engines to respond with power as it does in normal autopilot throttle control. Instead, the engines slowly rolled back, and the aircraft’s speed decayed. Minutes later, Air France 447 pancaked into the Atlantic, fully stalled.
Identifying and Placing the Correct Talent
Correctly preparing human operators requires not only determining the maturity of the system but also differentiating between tactical and strategic forms of AI. In tactical applications, like airplanes or missile defense systems, timelines may be compressed beyond human reaction times, forcing the human to give full trust to a system and allow it to operate autonomously. In strategic or operational situations, by contrast, AI is attempting to derive adversary intent which encompasses broader timelines and more ambiguous data. As a result, analysts who depend on an AI’s output need to be familiar with its internal workings in order take advantage of its superior data processing and pattern-finding capabilities.
Consider the tactical applications of AI in air-to-air combat. Drones, for example, may operate in semi-autonomous or fully autonomous modes. In these situations, human operators must exercise control restraint, known as neglect benevolence, to allow their AI wingmen to function without interference. In piloted aircraft, AI pilot assist programs may be providing turn-by-turn queues to the pilot to defeat an incoming threat, not unlike turn-by-turn directions given by the Waze application to car drivers. Sensors around the fighter aircraft detect infrared, optical, and electromagnetic signatures, calculate the direction of arrival and guidance mode of the threat, and advise the pilot on the best course of action. In some cases, the AI pilot may even take control of the aircraft if human reaction time is too slow, as with the automatic ground collision avoidance systems. When timelines are compressed and the type of relevant data is narrow, human operators do not need to be as familiar with the system’s behavior, especially once its proven or certified. Without the luxury of time to judge or second-guess AI behavior, they simply need to know and trust its capabilities.
However, the requirements will be different as AI gradually begins to play a bigger role in strategic processes like intelligence collection and analysis. When AI is being used to aggregate a wider swath of seemingly disparate data, understanding its approach is crucial to evaluating its output. Consider the following scenario: An AI monitoring system scans hundreds of refinery maintenance bulletins and notices that several state-controlled oil companies in a hostile country announce plans to shut down refineries for “routine maintenance” during a particular period. Then, going through thousands of cargo manifests, it discovers that a number of outbound oil tankers from that country have experienced delays in loading their cargo. The AI then reports that the nation in question is creating the conditions for economic blackmail. At this point, a human analyst could best assess this conclusion if they knew what kinds of delays the system had identified, how unusual these forms of delays were, and whether there were other political or environmental factors that might explain them.
Next Steps
With untrained operators, the force-multiplying effects of AI can be negated by the very people they are designed to aid. To avoid this, algorithm-dominated warfare requires updates to the way the military sifts and sorts its talent.
Tests like the Navy’s Aviation Selection Test Battery, the Air Force’s Officer Qualification Test, or the universal Armed Services Vocational Aptitude Battery rate a candidate’s performance in a range of subject areas. With machines replacing certain kinds of human expertise, the military needs to screen for new skills, specifically the ability to understand machine systems, processes, and programming. Changing entry exams to test for data interpretation skills and an ability to understand machine logic would be a valuable first step. Google’s Developers certification or Amazon’s Web Services certification offer useful models that the military could adapt. The military should also reward recruits and service members for completing training in related fields from already-available venues such as massive open online courses.
For those already in the service, the Secretary of Defense should promote relevant skills by prioritizing competitive selection for courses specializing in understanding AI systems. Existing examples include Stanford University’s Symbolic Systems Program, the Massachusetts’s Institute of Technology AI Accelerator course, and the Naval Postgraduate School’s “Harnessing AI” course. The military could also develop new programs based out of institutions like the Naval Community College or the Naval Postgraduate School and build partnerships with civilian institutions that already offer high-quality education in artificial intelligence. Incorporating AI literacy into professional military education courses and offering incentives to take AI electives would help as well. The Air Force’s computer language initiative, now reflected in Section 241 of the 2021 National Defense Authorization Act, represents an important first step. Nascent efforts across the services need to be scaled up to offer commercially relevant professional learning opportunities at all points during the service member’s career.
Artificial intelligence is rapidly disrupting traditional analysis and becoming a force multiplier for humans, allowing them to focus on orchestration rather than the minutia of rote and repetitive tasks. AI may also displace some current specializations, freeing people for roles that are better suited for humans. Understanding Kasparov’s Law can help the military cultivate the right talent to fully take advantage of this shift.
Trevor Phillips-Levine is a naval aviator and the Navy’s Joint Close Air Support branch officer. He has co-authored several articles regarding autonomous or remotely piloted platforms, publishing with the Center for International Maritime Security, U.S. Naval Institute Proceedings magazine, and Modern Warfare Institute. He can be reached on LinkedIn or Twitter.
Michael Kanaan is a Chief of Staff of the United States Air Force fellow at Harvard Kennedy School. He is also the author of T-Minus AI: Humanity’s Countdown to Artificial Intelligence and the New Pursuit of Global Power. You can find him on LinkedIn and Twitter.
Dylan Phillips-Levine is a naval aviator and a senior editor for the Center for International Maritime Security.
Walker D. Mills is a Marine infantry officer currently serving as an exchange officer at the Colombian Naval Academy in Cartagena, Colombia. He is also a nonresident fellow at the Brute Krulak Center for Innovation and Modern War and a nonresident fellow with the Irregular Warfare Initiative. He has written numerous articles for publications like War on the Rocks, Proceedings, and the Marine Corps Gazette.
Noah “Spool” Spataro is a division chief working Joint All Domain Command and Control assessments on the Joint Staff. His experiences traverse dual-use technology transition and requirements, standup and command of a remotely piloted aircraft squadron, and aviation command and control. He is a distinguished graduate of National Defense University’s College of Information and Cyberspace.
The positions expressed here are those of the authors and do not represent those of the Department of Defense or any part of the U.S. government.
Image: Public Domain”