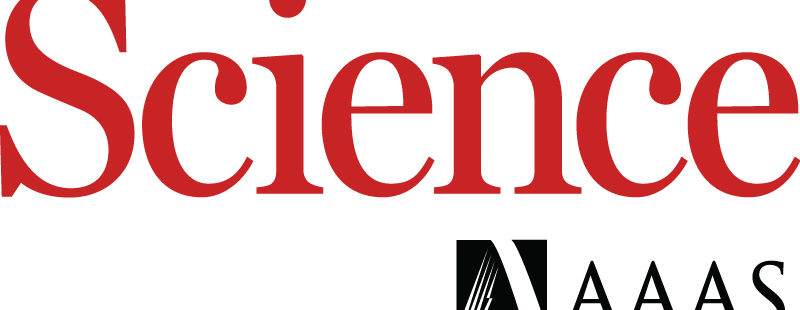
by Garry Kasparov
READ ORIGINAL ARTICLE AT SCIENCE MAGAZINE
The recent world chess championship saw Magnus Carlsen defend his title against Fabiano Caruana. But it was not a contest between the two strongest chess players on the planet, only the strongest humans. Soon after I lost my rematch against IBM’s Deep Blue in 1997, the short window of human-machine chess competition slammed shut forever. Unlike humans, machines keep getting faster, and today a smartphone chess app can be stronger than Deep Blue. But as we see with the AlphaZero system (see pages 1118 and 1140), machine dominance has not ended the historical role of chess as a laboratory of cognition.
Much as the Drosophila melanogaster fruit fly became a model organism for geneticists, chess became a Drosophila of reasoning. In the late 19th century, Alfred Binet hoped that understanding why certain people excelled at chess would unlock secrets of human thought. Sixty years later, Alan Turing wondered if a chess-playing machine might illuminate, in the words of Norbert Wiener, “whether this sort of ability represents an essential difference between the potentialities of the machine and the mind.”
Much as airplanes don’t flap their wings like birds, machines don’t generate chess moves like humans do. Early programs that attempted it were weak. Success came with the “minimax” algorithm and Moore’s law, not with the ineffable human combination of pattern recognition and visualization. This prosaic formula dismayed the artificial intelligence (AI) crowd, who realized that profound computational insights were not required to produce a machine capable of defeating the world champion.
But now the chess fruit fly is back under the microscope. Based on a generic game-playing algorithm, AlphaZero incorporates deep learning and other AI techniques like Monte Carlo tree search to play against itself to generate its own chess knowledge. Unlike top traditional programs like Stockfish and Fritz, which employ many preset evaluation functions as well as massive libraries of opening and endgame moves, AlphaZero starts out knowing only the rules of chess, with no embedded human strategies. In just a few hours, it plays more games against itself than have been recorded in human chess history. It teaches itself the best way to play, reevaluating such fundamental concepts as the relative values of the pieces. It quickly becomes strong enough to defeat the best chess-playing entities in the world, winning 28, drawing 72, and losing none in a victory over Stockfish.
I admit that I was pleased to see that AlphaZero had a dynamic, open style like my own. The conventional wisdom was that machines would approach perfection with endless dry maneuvering, usually leading to drawn games. But in my observation, AlphaZero prioritizes piece activity over material, preferring positions that to my eye looked risky and aggressive. Programs usually reflect priorities and prejudices of programmers, but because AlphaZero programs itself, I would say that its style reflects the truth. This superior understanding allowed it to outclass the world’s top traditional program despite calculating far fewer positions per second. It’s the embodiment of the cliché, “work smarter, not harder.”
AlphaZero shows us that machines can be the experts, not merely expert tools. Explainability is still an issue—it’s not going to put chess coaches out of business just yet. But the knowledge it generates is information we can all learn from. Alpha-Zero is surpassing us in a profound and useful way, a model that may be duplicated on any other task or field where virtual knowledge can be generated.
Machine learning systems aren’t perfect, even at a closed system like chess. There will be cases where an AI will fail to detect exceptions to their rules. Therefore, we must work together, to combine our strengths. I know better than most people what it’s like to compete against a machine. Instead of raging against them, it’s better if we’re all on the same side.